Infrared small moving target detection algorithm based on joint spatio-temporal sparse recovery
-
摘要: 提出了一种基于空时联合稀疏重构的红外小弱运动目标检测算法。通过学习序列图像内容而构建的空时联合字典能同时刻画目标或背景的形态特征和运动信息;利用多元高斯运动模式从空时联合字典中提取出目标空时字典和背景空时字典,目标空时过完备字典描述移动的目标,背景空时过完备字典表征背景噪声。将连续多帧图像在空时联合字典上进行稀疏分解,然后分别利用目标空时字典和背景空时字典中的最大稀疏系数及其空时原子重构信号,获取重构残余能量差异来区分目标和背景。试验结果表明,由同源的空时字典重构的残余能量小,而由异构的空时字典恢复的残余能量大,该方法不仅能提高序列信号表示的稀疏度,还能有效提高小运动目标的探测能力。Abstract: A dim small moving target detection algorithm based on joint spatio-temporal sparse recovery is proposed in this paper. A spatio-temporal over-complete dictionary is firstly trained from infrared image sequence, and it can characterize not only motion information but also morphological feature. In the spatio-temporal over-complete dictionary, the spatio-temporal atom are then classified as target spatio-temporal atoms building target spatio-temporal over-complete dictionary, which describes moving target, and background spatio-temporal atoms constructing background spatio-temporal over-complete dictionary, which embeds background clutter. Infrared image sequence is decomposed on the union of target spatio-temporal over-complete dictionary and background spatio-temporal over-complete dictionary. The residuals after decomposing and reconstruction by the target spatio-temporal over-complete dictionary and background over-complete dictionary differ very distinctly, and they are then adopted to decide the signal is from target or background. Some experiments are conducted and the experimental results show that the residual reconstructed by its homologous spatio-temporal over-complete dictionary is very little, yet the residual recovered by its heterogonous spatio-temporal over-complete dictionary is quite large. This proposed approach could not only improve the sparsity more efficiently, but also enhance the target detection performance more effectively.
-
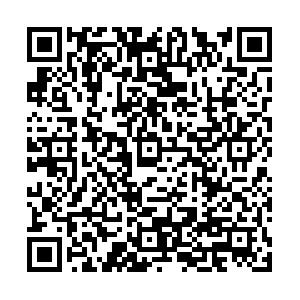
计量
- 文章访问数: 1073
- HTML全文浏览量: 281
- PDF下载量: 198
- 被引次数: 0