Dim moving target tracking algorithm based on particle sparse representation
-
摘要: 评估每个粒子的重要性是确保粒子滤波法跟踪目标准确性的重要因素。针对背景杂波和噪声干扰形成的大量虚警导致小弱目标跟踪识别的随机性和不确定性问题, 提出了一种基于粒子区别性稀疏表征的小弱目标跟踪方法。该方法根据红外图像信号自适应构建分类超完备字典, 即反映目标信号特征的目标字典和表示背景杂波的背景字典, 有利于突出目标粒子和背景粒子在联合分类字典的稀疏表征差异程度;建立基于目标粒子和背景粒子稀疏重构残差差异性的粒子滤波观测模型, 采用随机估计法对字典子空间进行在线更新, 实现对目标状态估计与跟踪。理论分析和试验结果表明, 该方法增强了随机粒子的状态估计能力, 提升了粒子稀疏表征对小弱运动目标的适应能力和跟踪识别准确度。Abstract: Assessing the importance of every particle is the key factor to ensure the accuracy of the dim target tracking based on particle filter. A small dim target tracking algorithm based on particle sparse discriminative representation is proposed in this paper to cope with the issue of the uncertainty of moving target tracking. An adaptive discriminative over-complete dictionary is trained and constructed according to infrared image, wherein the target dictionary describes the target signals character and the background dictionary embeds the background clutter. It is helpful to highlight the difference between the target particle and the background particle in the adaptive discriminative over-complete dictionary. The importance of every particle is constructed based on the significant residual difference between target particle and background particle, and then the observation model of particle filter is estimated to track target state. Meanwhile, the subspace of the over-complete dictionary is updated online by the stochastic estimation algorithm. Some experiments were carried out and the experimental results show this proposed approach could not only enhance the target state estimation ability of particle, but also improve the adaptive ability and target recognition accuracy of small dim moving target.
-
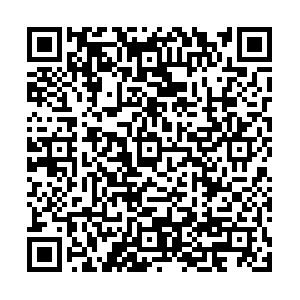
计量
- 文章访问数: 1119
- HTML全文浏览量: 228
- PDF下载量: 430
- 被引次数: 0