Adaptive kernel collaborative representation anomaly detection for hyperspectral imagery
-
摘要: 针对高光谱图像目标检测问题,提出了一种基于自适应核联合表示的高光谱图像异常检测方法。相比于稀疏表示强调系数向量的稀疏性,基于联合表示的检测算法更强调背景字典中每一原子对联合表示的贡献,并且系数求解更加简单直接;在基于向量2-范数极小化问题求解联合表示系数时,引入相似性正则化矩阵与和为1的条件对系数进行约束,以增强算法的稳定性和分辨力;进而将联合表示检测算法扩展到核空间,利用高光谱图像的局部统计特性进行核参数自适应选取,增强了核参数的局部适应性。为验证该方法的有效性,采用一幅实测高光谱图像中的两个感兴趣区域进行仿真实验,并与传统异常检测方法的检测结果进行对比分析,结果表明该方法具有较传统异常检测方法更出色的检测效果。Abstract: An adaptive kernel collaborative representation based anomaly detector for hyperspectral imagery was proposed in view of target detection. Although the sparse representation considers the sparsity of weighted vector, the collaborative representation puts more emphasis on each atoms contribution to the linear combination. A comparability weighted regularization matrix and sum-to-one constraint were employed to reinforce the stability and separability. Then, the kernel parameter was adaptively estimated by using local statistics to improve its own local adaption. The proposed AKCRD algorithm was applied to two hyperspectral datasets comparing with other algorithms such as RX, KRX, SVDD, CRD and KCRD. The simulation results show that the proposed algorithm has better detection performance.
期刊类型引用(9)
1. 冯现永. 基于小波分析的图书馆电子阅览设备故障检测方法. 自动化与仪器仪表. 2021(02): 46-49 . 百度学术
2. 杨敬,贾召会,龚梦彤,蔡伟,刘佳豪,王轩,樊艳春. 基于多信号流图的亚跨超声速风洞故障诊断方法. 计算机测量与控制. 2021(08): 67-71 . 百度学术
3. 苏健,吴秀. 半导体激光宫颈糜烂治疗仪故障在线监测研究. 自动化与仪器仪表. 2020(01): 146-149 . 百度学术
4. 冯晓晖. 基于激光定位的非线性故障检测系统. 激光杂志. 2020(01): 81-85 . 百度学术
5. 黄将. 数据中心光纤通信网络传输非平稳数据无损检测技术研究. 激光杂志. 2019(04): 131-135 . 百度学术
6. 邵健,庄泽旭. 医用激光相机设备故障快速检测系统设计. 激光杂志. 2019(05): 104-107 . 百度学术
7. 王艳阁. 光纤传感网络链路故障自修复系统设计. 激光杂志. 2018(03): 172-175 . 百度学术
8. 黄燕. 复杂复印机故障信号的检测与提取. 现代电子技术. 2018(22): 103-105+109 . 百度学术
9. 吴华芹. 基于机器学习的光纤故障数据信息快速排除方法. 激光杂志. 2018(12): 160-165 . 百度学术
其他类型引用(2)
-
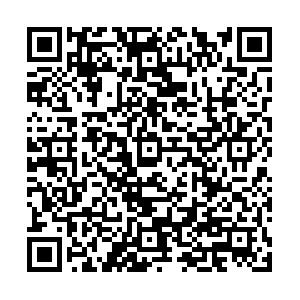
计量
- 文章访问数: 1015
- HTML全文浏览量: 239
- PDF下载量: 394
- 被引次数: 11