Deep learning phase inversion technique for single frame image based on Walsh function modulation
-
摘要: 远场波前反演存在简并态,复原波前容易出现多解问题。相较于传统的迭代算法,结合了相位调制与深度学习的相位反演方法不仅显著降低了计算复杂度,还可有效地解决多解问题。这种方法实时性强,结构简洁,显示出其独特的优势。使用不同的沃尔什函数(Walsh)对相位进行调制,采取深度学习的方法训练卷积神经网络从调制后单帧远场强度图中获得4~30阶Zernike系数从而复原出原始波前,解决了相位反演多解问题。对于3~15 cm大气相干长度的湍流像差的残差波前,其RMS与原始波前RMS的比值可达7.8%。深入研究了Zernike阶数、随机噪声、遮挡以及强度图分辨率等多种因素对波前复原精度的影响。研究结果表明,这种基于深度学习的相位反演方法在复杂的环境中展现出了良好的鲁棒性。Abstract: The far-field wavefront inversion exhibits degeneracy states, leading to the problem of encountering multiple solutions when recovering the wavefront. In comparison to traditional iterative algorithms, the combination of phase modulation and deep learning in the phase inversion method not only significantly reduces computational complexity but also effectively solves multi-solution problems. This method possesses strong real-time capabilities and a simple structure, showcasing its unique advantages. In this paper, different Walsh functions are used to modulate the phase, and a deep learning approach is taken to train a convolutional neural network to obtain the 4th-30th order Zernike coefficients from the modulated single-frame far-field intensity maps so as to recover the original wavefront, which solves the problem of multiple solutions of phase inversion. For the residual wavefront of the turbulent aberration of 3-15 cm atmospheric coherence length, the ratio of its RMS to the RMS of the original wavefront can reach 7.8%. In addition, this paper also deeply investigates the effects of various factors such as Zernike order, random noise, occlusion, and intensity map resolution on the wavefront recovery accuracy. The results show that this deep learning-based phase inversion method exhibits good robustness in complex environments.
-
Key words:
- Phase inversion /
- Zernike coefficient /
- Phase modulation /
- Deep learning /
- Walsh function
-
表 1 不同Walsh函数调制样本训练网络测试结果对比
Table 1. Comparison of test results of different Walsh function modulated sample training networks
Walsh function ResNet18 RMSE/μm ResNet34 RMSE/μm ResNet50 RMSE/μm ResNet101 RMSE/μm W12 0.0115 0.0105 0.0080 0.0069 W15 0.0111 0.0092 0.0062 0.0067 W3 0.0103 0.0087 0.0055 0.0054 表 2 不同Zernike阶数波前的RMS比值平均值
Table 2. Average RMS ratio with Zernike order wavefront
different Zernike levels average RMS ratio/% Zernike4~10 9.6 Zernike4~15 8.6 Zernike4~20 8.5 Zernike4~25 8.4 Zernike4~30 7.8 表 3 不同像素远场强度图的RMS比值平均值
Table 3. Average RMS ratio of far-field intensity map with different pixels
intensity image pixels average RMS ratio/% 256×256 7.8 512×512 10.2 1024×1024 12.9 表 4 噪声样本在不同模型下的RMS比值平均值
Table 4. Average RMS ratio of noise samples under different models
test data set samples for training purposes average RMS ratio of residual wavefront
to original wavefront/%256×256 with random noise samples 350 000 normal sample invalid 256×256 with random noise samples 350 000 normal sample+ 35 W noise sample 6.5 -
[1] Gerchberg R W, Saxton W O. A practical algorithm for the determination of phase from image and diffraction plane pictures[J]. Optik, 1972, 35(2): 237-246. [2] Gonsalves R A. Phase retrieval and diversity in adaptive optics[J]. Optical Engineering, 1982, 21: 215829. [3] 张杏云, 罗芳琳, 李楠, 等. 相位差波前探测与图像重建[J]. 强激光与粒子束, 2021, 33:081010Zhang Xingyun, Luo Fanglin, Li Nan, et al. Phase diversity wavefront sensing and image reconstruction[J]. High Power Laser and Particle Beams, 2021, 33: 081010 [4] 张全. 相位差算法的并行化分析与实现[D]. 成都: 电子科技大学, 2015: 66-98Zhang Quan. Parallel analysis and implementation of phase diversity[D]. Chengdu: University of Electronic Science and Technology of China, 2015: 66-98 [5] 孔庆峰. 基于单帧焦面图像的波前相位反演方法研究[D]. 成都: 电子科技大学, 2019: 35-44Kong Qingfeng. Research on wavefront phase retrieval method based on single frame focal plane image[D]. Chengdu: University of Electronic Science and Technology of China, 2019: 35-44 [6] 孔庆峰, 王帅, 杨平, 等. 基于Walsh函数调制的单帧远场波前反演方法[J]. 光电工程, 2020, 47:190323Kong Qingfeng, Wang Shuai, Yang Ping, et al. Single-frame far-field wavefront retrieval method based on Walsh function modulation[J]. Opto-electronic Engineering, 2020, 47: 190323 [7] Xin Qi, Ju Guohao, Zhang Chunyue, et al. Object-independent image-based wavefront sensing approach using phase diversity images and deep learning[J]. Optics Express, 2019, 27(18): 26102-26119. doi: 10.1364/OE.27.026102 [8] 张阳, 何宇龙, 宁禹, 等. 远场光斑反演波前相位的深度学习方法[J]. 红外与激光工程, 2021, 50:20200363 doi: 10.3788/IRLA20200363Zhang Yang, He Yulong, Ning Yu, et al. Method of inverting wavefront phase from far-field spot based on deep learning[J]. Infrared and Laser Engineering, 2021, 50: 20200363 doi: 10.3788/IRLA20200363 [9] 史宗佳, 向振佼, 杜应磊, 等. 基于远场信息和卷积神经网络的波前重构方法[J]. 强激光与粒子束, 2021, 33:081011Shi Zongjia, Xiang Zhenjiao, Du Yinglei, et al. Wavefront reconstruction method based on far-field information and convolutional neural network[J]. High Power Laser and Particle Beams, 2021, 33: 081011 [10] 周静, 张晓芳, 赵延庚. 一种基于图像融合和卷积神经网络的相位恢复方法[J]. 物理学报, 2021, 70:054201 doi: 10.7498/aps.70.20201362Zhou Jing, Zhang Xiaofang, Zhao Yangeng. Phase retrieval wavefront sensing based on image fusion and convolutional neural network[J]. Acta Physica Sinica, 2021, 70: 054201 doi: 10.7498/aps.70.20201362 [11] 邱学晶. 基于四象限离散相位调制的单帧图像深度学习相位反演算法研究[D]. 成都: 中国科学院大学, 2021: 28-30Qiu Xuejing. Research on single frame deep learning phase retrieval method based on four-quadrant discrete phase modulation[D]. Chengdu: University of Chinese Academy of Sciences, 2021: 28-30 [12] McGlamery B L. Computer simulation studies of compensation of turbulence degraded images[J]. Journal of the Optical Society of America, 1976, 66(2): 225-233. [13] 杨海波, 许宏. 基于功率谱反演法的大气湍流相位屏数值模拟[J]. 光电技术应用, 2019, 34(4):73-76Yang Haibo, Xu Hong. Numerical simulation of atmospheric turbulence phase screen based on power spectrum inversion method[J]. Electro-Optic Technology Application, 2019, 34(4): 73-76 [14] Walsh J L. A closed set of normal orthogonal functions[J]. American Journal of Mathematics, 1923, 45(1): 5-24. doi: 10.2307/2387224 [15] Wang S, Yang P, Ao M W, et al. Wavefront sensing by means of binary intensity modulation[J]. Applied Optics, 2014, 53(35): 8342-8349. doi: 10.1364/AO.53.008342 [16] He Kaiming, Zhang Xiangyu, Ren Shaoqing, et al. Deep residual learning for image recognition[C]//2016 IEEE Conference on Computer Vision and Pattern Recognition (CVPR). 2016: 770-778. -
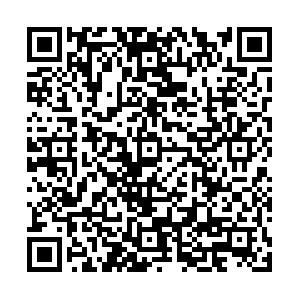
计量
- 文章访问数: 18
- HTML全文浏览量: 10
- PDF下载量: 0
- 被引次数: 0